一、讲座时间
2025年9月5日(周四)下午15:40-17:00
二、讲座内容
主题:用于计算机视觉和不确定性的机器学习。
Model calibration measures how well the confidence of a model’s predictions matches its actual accuracy. This is important for determining how trustworthy the model is. Many existing methods for binary classification (where the model predicts one of two outcomes) tend to be too confident in their predictions when they don’t properly regulate confidence.
To address this, we propose a method called Self-Calibrating Vicinal Risk Minimization (SCVRM) to improve the calibration of Deep Neural Networks (DNNs). This method looks at the area around labeled data and assigns lower confidence to images that are further from the labeled examples. We show that for logistic regression (a common type of model), SCVRM works like regular Vicinal Risk Minimization but adds a term that penalizes over-confident predictions.
In practice, SCVRM uses Monte Carlo sampling, which means it generates additional training data by creating new, slightly different images and assigning them labels with varying confidence. Our experiments show that SCVRM can significantly improve model calibration across various classification tasks, even when the data comes from different distributions than the training data.
三、主讲人
澳大利亚国立大学工程学院Nick Barnes院长
四、讲座地点
中南大学新校区信息楼3楼智空间
五、报名方式
请同学们扫描下方二维码或填写链接中的信息完成报名。
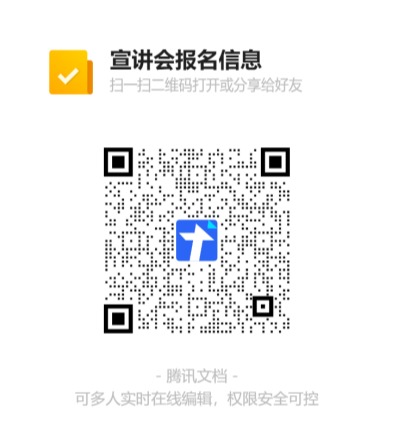
【腾讯文档】
https://docs.qq.com/form/page/DZVFvZkZhaEluSllX
六、联系与咨询
联系人:文老师、周老师
电话:88877945
邮箱:ied-csu@csu.edu.cn